Introducing the Describe Anything Model (DAM)
The Describe Anything Model (DAM) is a powerful multimodal large language model that can generate detailed descriptions for specific regions in images or videos. Users can specify regions using points, boxes, scribbles, or masks, and DAM will provide rich, contextual descriptions of those regions. Watch our intro video below to learn more about DAM.
Detailed Localized Captioning (DLC)
Detailed Localized Captioning (DLC) is the task of generating comprehensive and context-aware descriptions of specific regions within an image. Unlike traditional image captioning, which summarizes the entire scene in broad strokes, DLC dives deeper into the finer details of a user-specified area. The goal is to capture not only the object's name or category but also subtle attributes such as texture, color patterns, shape, notable parts, and any visually distinctive features.
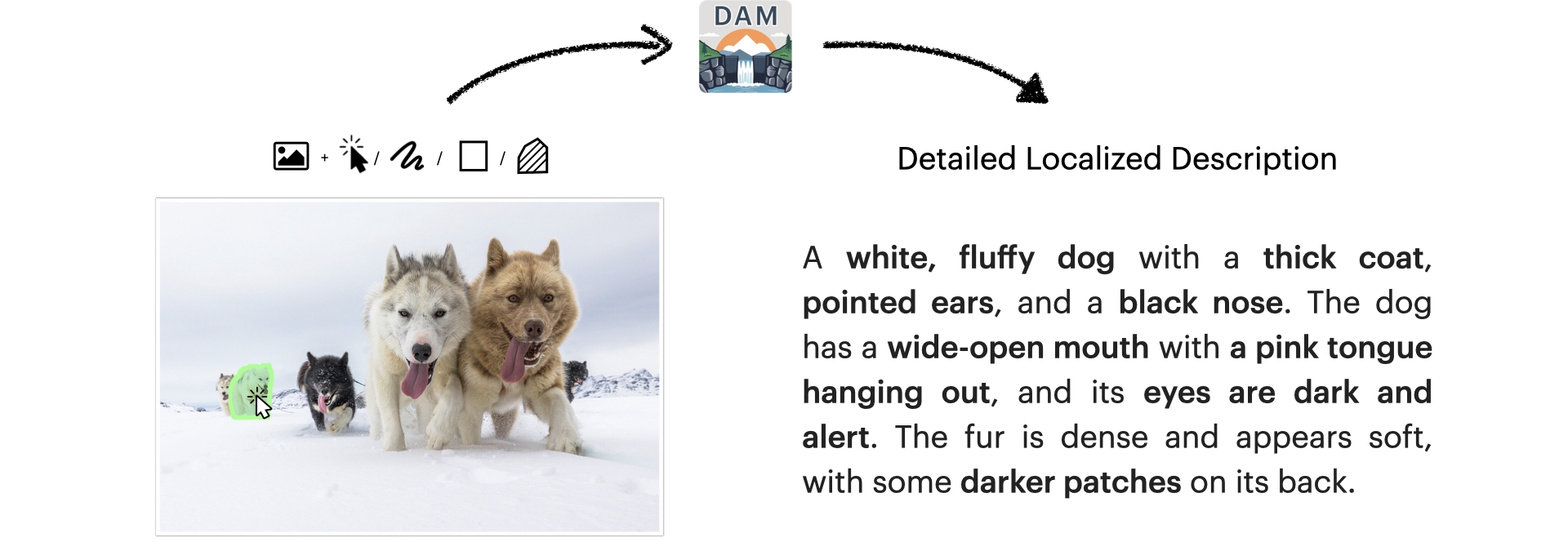
Describe Anything Model (DAM) performs detailed localized captioning (DLC), generating detailed and localized descriptions for user-specified regions within images. DAM accepts various user inputs for region specification, including clicks, scribbles, boxes, and masks.
(Image credit: Markus Trienke (CC BY-SA 2.0))
DLC extends naturally to videos by describing how a specified region's appearance and context change over time. Models must track the target across frames, capturing evolving attributes, interactions, and subtle transformations.
Describe Anything Model (DAM) can also perform detailed localized video captioning, describing how a specified region changes over time. For localized video descriptions, specifying the region on only a single frame is sufficient. See our paper for full descriptions.
(Video credit: MOSE Dataset (CC BY-NC-SA 4.0))
Highly Detailed Image and Video Captioning
Our method excels at producing detailed descriptions of objects in both images and videos. By balancing the clarity of a focal region with global context, the model can highlight subtle features—like intricate patterns or changing textures—far beyond what general image-level captioning provides.
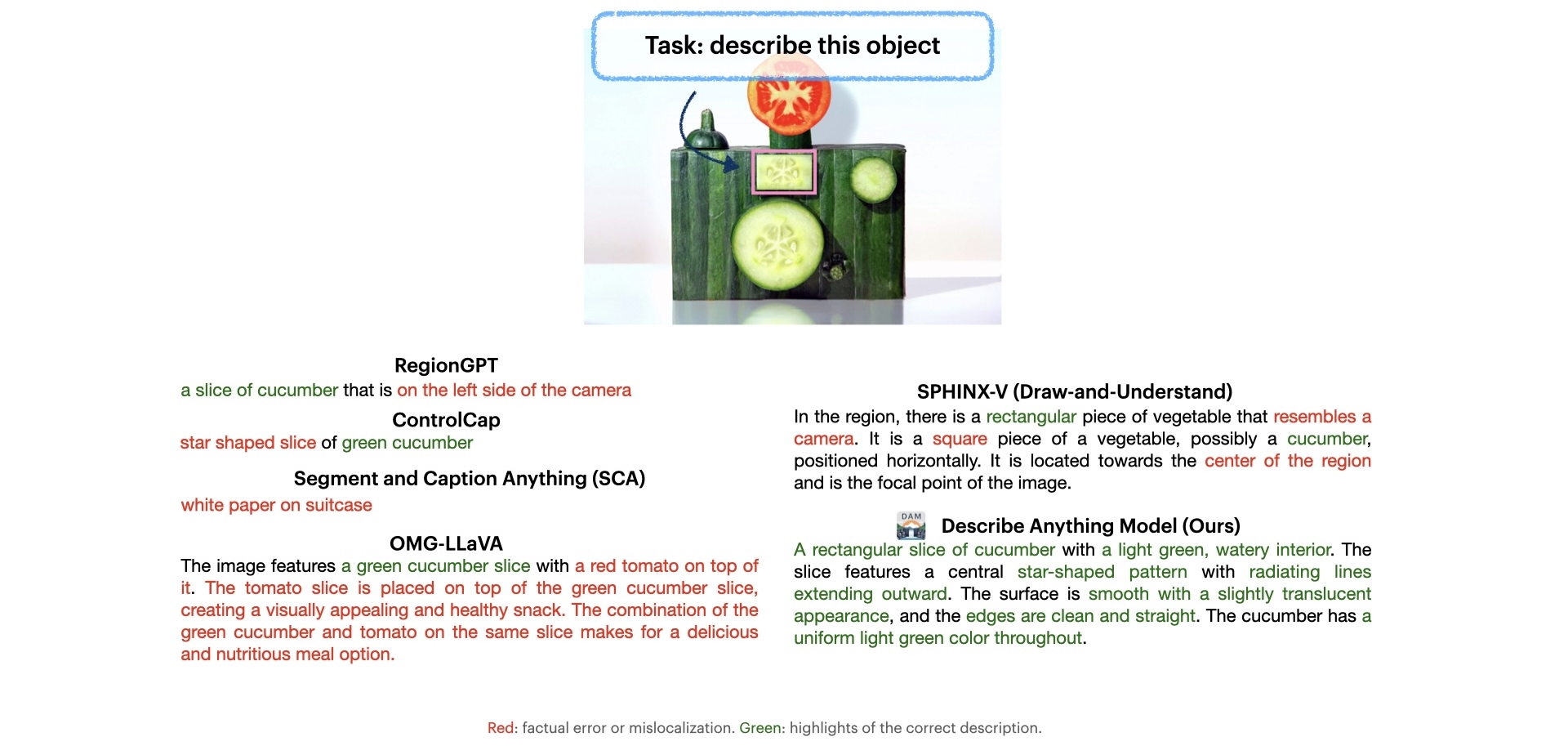
Compared to prior works, the description from our Describe Anything Model (DAM) is more detailed and accurate. (Image credit: SAM Materials (CC BY-SA 4.0))
Instruction-controlled Captioning
Users can guide our model to produce descriptions of varying detail and style. Whether a brief summary or a long, intricate narrative is needed, the model can adapt its output. This flexibility benefits diverse use cases, from rapid labeling tasks to in-depth expert analyses.
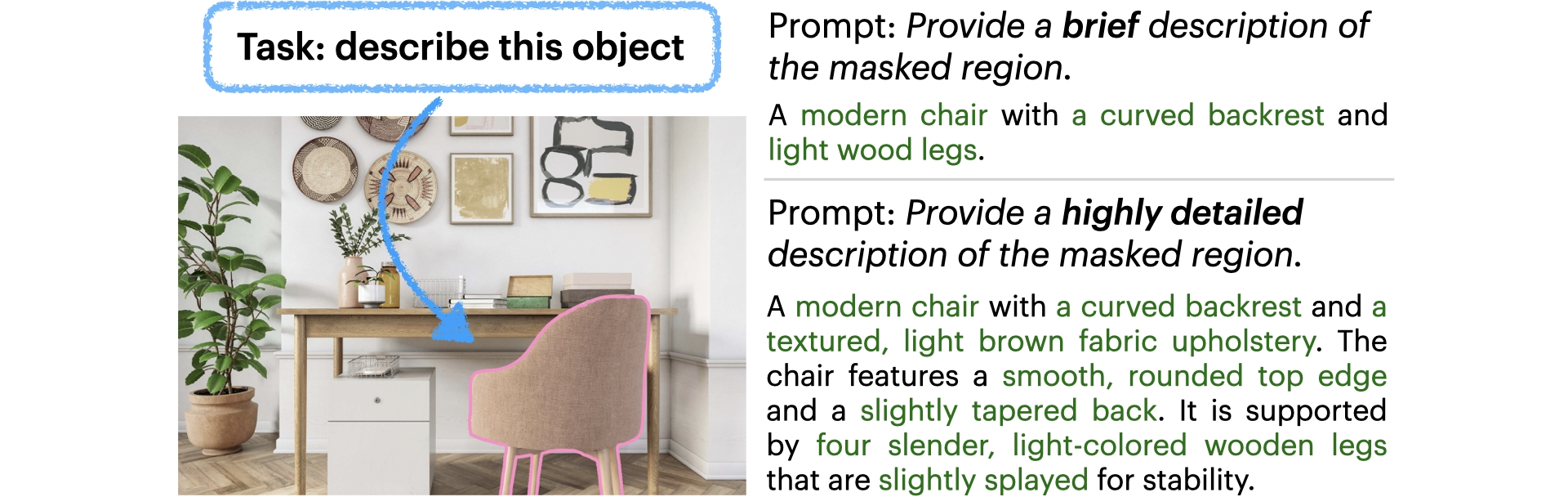
Describe Anything Model (DAM) can generate descriptions of varying detail and style according to user instructions.
(Image credit: SAM Materials (CC BY-SA 4.0))
Zero-shot Regional QA
Beyond descriptions, our model can answer questions about a specified region without extra training data. Users can ask about the region's attributes, and the model draws on its localized understanding to provide accurate, context-driven answers. This capability enhances natural, interactive use cases.
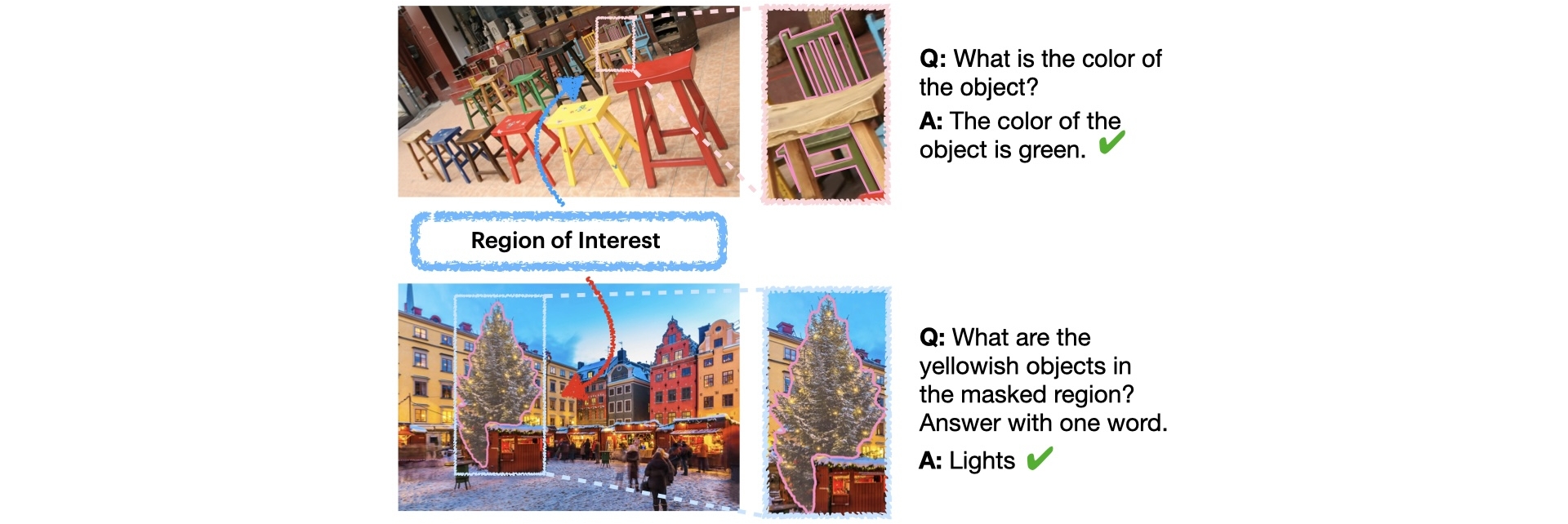
Architecture of Describe Anything Model (DAM)
Our architecture uses a "Focal Prompt" to provide both the full image and a zoomed-in view of the target region. This approach ensures the model sees fine details while retaining global context. The result is detailed, accurate captions that reflect both the bigger picture and the smallest nuances.
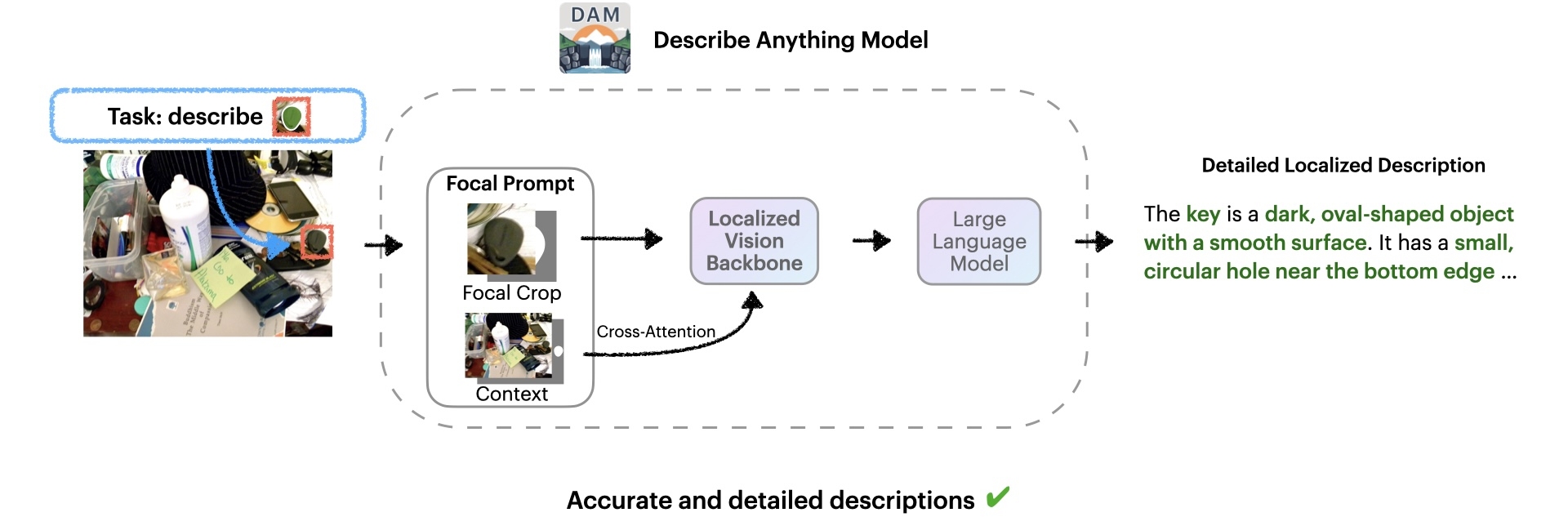
Image credit: Objects365 Dataset (CC BY 4.0)
We introduce a localized vision backbone that integrates global and focal features. Images and masks are aligned spatially, and gated cross-attention layers fuse detailed local cues with global context. New parameters are initialized to zero, preserving pre-trained capabilities. This design yields richer, more context-aware descriptions.
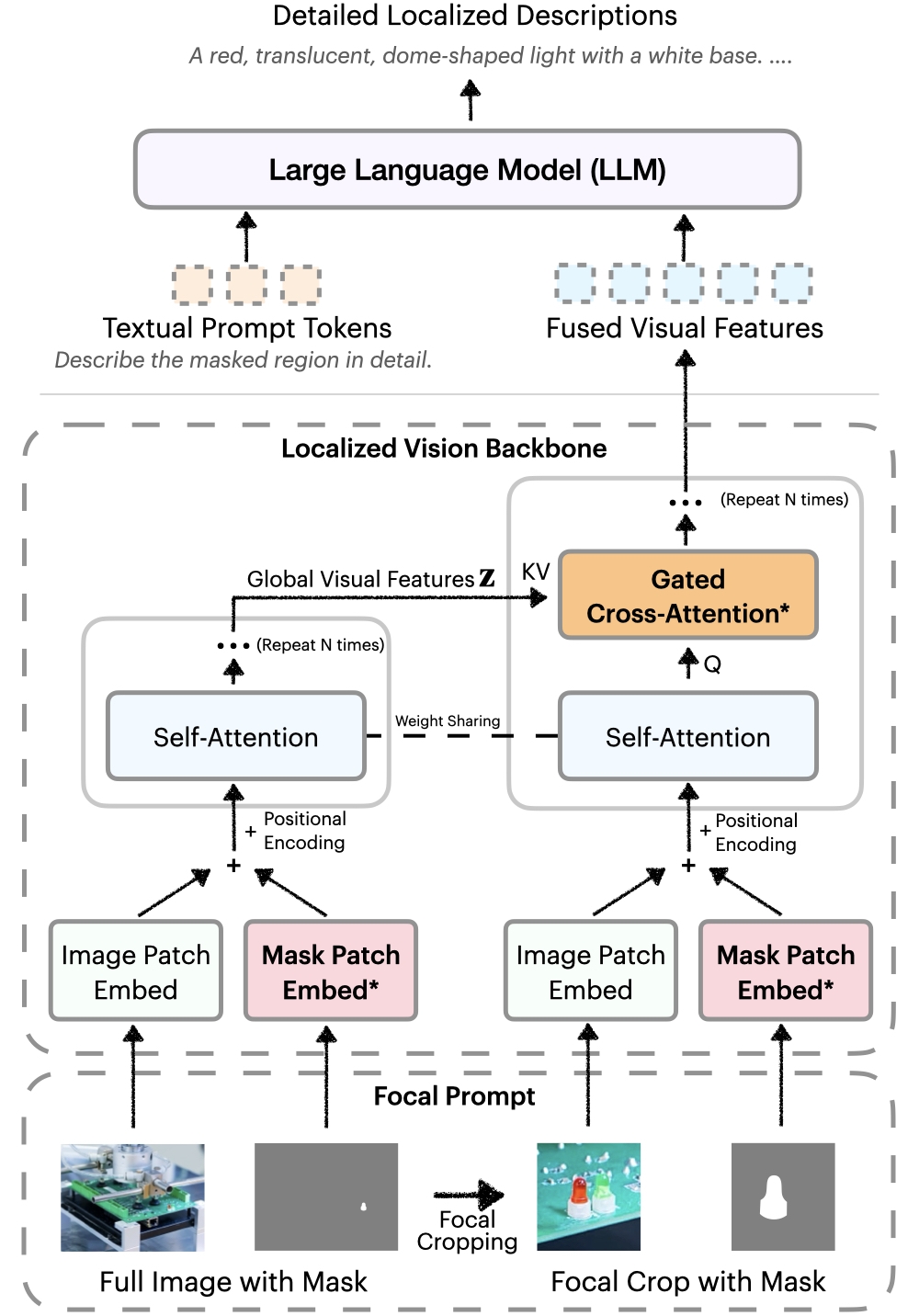
Semi-supervised Data Pipeline for Detailed Localized Captioning (DLC-SDP)
Because existing datasets lack detailed localized descriptions, we devised a two-stage pipeline. First, we use a VLM to expand short class labels from segmentation datasets into rich descriptions. Second, we apply self-training as a form of semi-supervised learning on unlabeled images, using our model to generate and refine new captions. This scalable approach builds large, high-quality training data without relying on extensive human annotation.
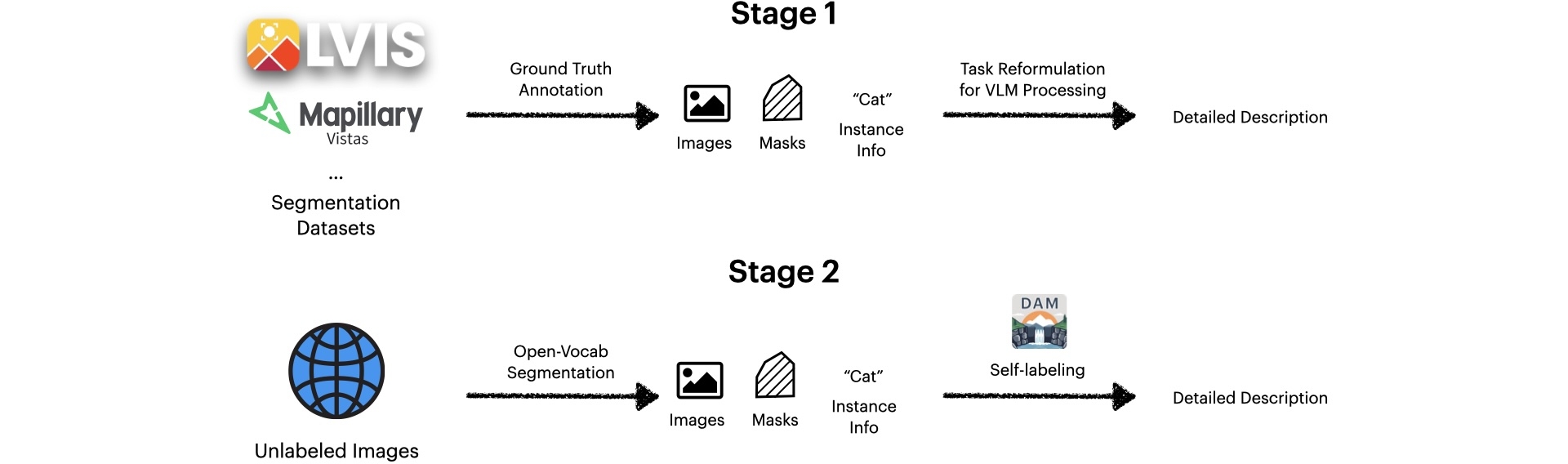
DLC-Bench: A Benchmark for Detailed Localized Captioning
We introduce DLC-Bench, a benchmark that uses an LLM-based judge to evaluate a model's region-based descriptions. Instead of relying on simple text overlap, DLC-Bench checks for correct details and the absence of errors. This offers a more accurate and human-like metric for measuring DLC performance.
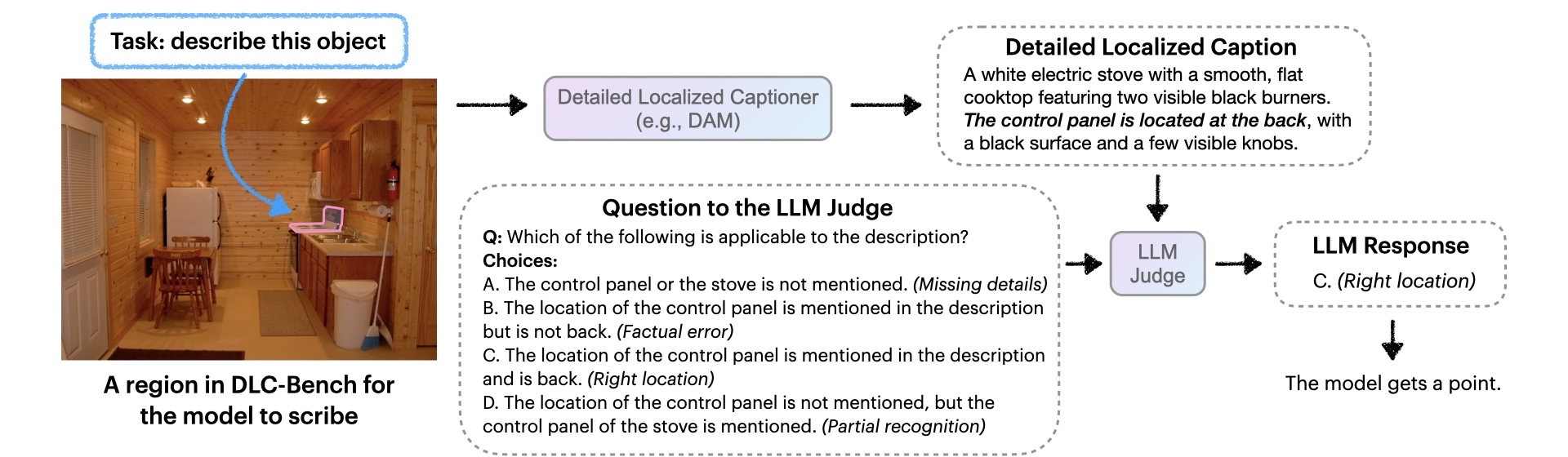
In DLC-Bench, a captioning model is prompted to describe a specified image region. The generated description is then evaluated by querying an LLM Judge. Points are assigned or deducted based on the LLM's response. The question we show is an example of positive questions.
(Image credit: Objects365 Dataset (CC BY 4.0))
Advantages of DAM, DLC-SDP, and DLC-Bench
Component | Previous Practice | Problem | Our Solution | Advantages |
---|---|---|---|---|
Describe Anything Model (DAM) | Extracting regional features from global image features | Regional details already lost in image feature extraction and not provided to the LLM | Providing focal prompt to proposed localized vision backbone | Detail-rich contextful features allowing for accurate, multi-granular localized descriptions |
SSL Data Pipeline (DLC-SDP) | Query a data curation VLM with referring boxes and global image captions | Imprecise referring to data curation model | Reframe the query into a mask-referred keyword expansion question | Leverage high-quality precise human annotated regional masks and keywords |
Fully supervised learning | Limited data with high annotation quality | Semi-supervised learning | Scalable to diverse web-scale unlabeled datasets | |
Benchmark (DLC-Bench) | Pred caption + reference GT caption → language-based similarity metrics or LLM scorer | Incorrect hallucination penalty for correct details not present in the reference caption | Pred caption + query for positive/negative attributes → LLM scorer | Accurate detail and hallucination assessment without relying on reference captions |
Comparison
On DLC-Bench, our model outperforms existing solutions by producing more detailed and accurate localized descriptions with less hallucination. It surpasses models trained for general image-level tasks and those designed for localized reasoning, setting a new standard for detailed, context-rich captioning.
Conclusion
Our Describe Anything Model (DAM) generates detailed descriptions for specified regions in images and videos, and can be utilized in various applications ranging from data annotation to serving as an intermediate component in downstream tasks. We plan to publicly release our code, models, data, and benchmark to support future research. We are excited to see the community explore the potential of detailed localized captioning. We hope this benchmark and model will serve as a useful resource for future research in this area.
Citation
If you use this work or find it helpful, please consider citing:
@article{lian2025describe, title={Describe Anything: Detailed Localized Image and Video Captioning}, author={Long Lian and Yifan Ding and Yunhao Ge and Sifei Liu and Hanzi Mao and Boyi Li and Marco Pavone and Ming-Yu Liu and Trevor Darrell and Adam Yala and Yin Cui}, journal={arXiv preprint arXiv:2504.16072}, year={2025} }